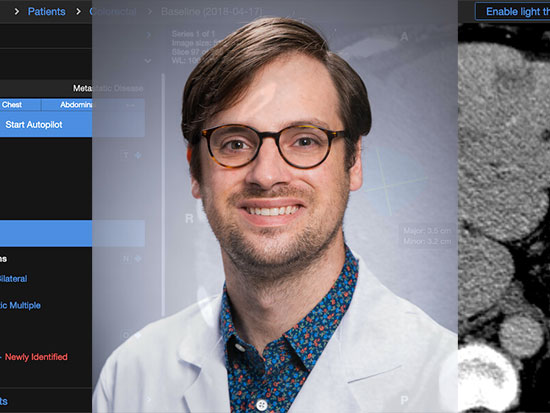
Radiology has an image problem. Specifically, the visual of a future where artificial intelligence (AI) algorithms have put radiologists out of work. In 2016, machine-learning pioneer Geoffrey Hinton unleashed this incendiary quote: “I think if you work as a radiologist, you are like the coyote that’s already over the edge of the cliff but hasn’t yet looked down,” Hinton said. “People should stop training radiologists now. It’s just completely obvious within five years deep learning is going to do better than radiologists …. It might be 10 years, but we’ve got plenty of radiologists already.”
In some ways, Hinton’s prediction has come true. Deep learning and other machine learning algorithms have flooded the field. As of early November 2022, there were 200 FDA-approved radiology AI algorithms ready for use, according to the American College of Radiology’s AI Central site. At UAB and some other academic medical centers, radiology faculty now use AI-enhanced tools as a routine part of care. Computer-aided detection and triage software can reduce turnaround time by automatically highlighting positive findings within images. Natural language processing tools, trained on millions of radiologists’ reports, can generate straightforward written conclusions based on findings notes that the physician can edit as needed. (See “Five ways radiologists are using AI.”)
At the same time, there is a global radiologist shortage, driven in part by overwork. “The amount of imaging is going up 5 percent per year, and we’re not training 5 percent more radiologists per year,” said Jordan Perchik, M.D., a fellow in the Department of Radiology at the UAB Heersink School of Medicine. (A 2021 study reported a 2.5 percent increase in diagnostic radiology trainees between 2010 and 2020, compared with a 34 percent increase in the number of adults over 65, a population that requires more imaging.) “The most commonly used AI tools,” Perchik said, “are ones that speed up scans, paradoxically increasing the workload for radiologists.”
The AI hype cycle in radiology has quieted somewhat as dramatic results from early studies have failed to pan out in real-world settings. But the potential benefits are too large to ignore, Perchik says, quoting a response to Hinton made by Stanford radiologist and AI pioneer Curtis Langlotz, M.D., Ph.D. “He said, ‘AI won’t replace radiologists, but radiologists who use AI will replace those who don’t,’” Perchik said.
AI Literacy Course 2022
Lectures from the 2022 edition of the AI Literacy Course, held in October 2022, with links to each lecture on the AI in Radiology Education YouTube channel.
- Introduction to the Artificial Intelligence Literacy Course
- Introduction to Medical AI Terms and Methods
- AI in Pediatric Imaging
- The Future of AI in Radiology
- Special Topics in AI: Federated Learning
- Potential Pitfalls of AI in MSK [Musculoskeletal] Research and Applications
- AI in Nuclear Medicine
- Economics and Ethics of AI
- AI Literacy Course: Week in Review
Increasingly, radiologists who want to know more about AI are getting their introduction from Perchik. In 2020, he began a series of lectures on the topic for his fellow UAB radiology trainees. The next year, residents from nine programs in the Southeast participated. By the October 2022 edition of Perchik’s AI Literacy Course, “we had 25 programs in 10 different countries participating,” he said. Aided by a grant from the Radiological Society of North America, Perchik now oversees AI in Radiology Education, the largest free resource for AI education for radiologists worldwide. “I wanted people to see that this is a tool that can help radiologists, not something to be feared,” Perchik said. (See a list of lectures at right and watch the 2022 lectures here.)
Another “sky is falling” moment
When Hinton said, “stop training radiologists” in 2016, Perchik was in his third year of medical school, and had just decided to specialize in radiology. At the time, a rash of AI studies demonstrating human or even superhuman performance at radiology tasks was all over the news. “That was a ‘sky is falling’ moment in radiology,” Perchik said. But as the son of a radiologist, Perchik knew this was not the first. “Since the early 1990s, just in my lifetime, there have been a few ‘sky is falling’ moments,” he said. “In the transition from film to digital systems, the fear was, ‘No one needs a radiologist now, because they can look at their own exams.’ Obviously, that didn’t happen.” Then there was the advent of computer-aided detection in mammography. “That is a rudimentary AI system,” Perchik said. “People were saying, ‘This is the end of breast imaging.’ But now that is one of the most in-demand specialties.”
A screenshot from the "AI in Nuclear Medicine" talk during the AI Literacy Course in 2022. Radiology has only expanded over that time, but radiologists did have to adapt to the new technologies and routines. “These experts were saying that human radiologists will be totally replaced by computers,” Perchik said. “That didn’t sit right with me. I started to learn more about AI — where it is strong and where it is weak.”
An AI “juggernaut”
After he began his radiology residency at UAB, Perchik met several new faculty members who were heavily engaged in AI research: Professor Srini Tridandapani, M.D., Associate Professor Houman Sotoudeh, M.D., and Professor Andrew Smith, M.D., Ph.D. “UAB has become this juggernaut of publications and AI research and entrepreneurship,” Perchik said. “I was impressed with the work they were doing and wanted to learn more.” Other radiology residents felt the same way, and Perchik asked program director and Associate Professor Jessica Zarzour, M.D., for permission to develop a weeklong curriculum on AI in radiology.
“You can find plenty of videos on YouTube and free courses on Coursera and elsewhere about AI and machine learning, but they are all from a computer science or hard science lens,” Perchik said. “I was interested in helping people get started with targeted lectures about the fundamentals of AI for radiology and how AI was being applied or could be applied in each radiology subspecialty. And I immediately had great interest and support from Dr. Zarzour and the rest of the department.”
About 75 percent of the participants have been residents, “but I’ve also found a lot of interest from practicing radiologists who never had that kind of training, and there has been a substantial increase in the number of medical students,” Perchik said.
“The program has grown more than I could ever have imagined.... We hope to build one of the hubs for AI education and scholarship throughout the United States and internationally.”
In addition to introductory lectures on AI concepts and lectures on using AI in each of radiology’s five subspecialties, Perchik adds new topics based on participant requests. In 2022, for example, Emory University’s Hari Trivedi, M.D., director of the university’s Healthcare Innovation and Translational Informatics Lab, discussed the economics and ethical considerations of AI algorithms. (See “Economics of AI for radiology.”) For the past two years, Smith’s talk “The Future of AI in Radiology” received the AI Literacy Course’s Most Impactful Lecture award. (See “Five ways radiologists are using AI.”)
International hub and local recruiting tool
Next year, Perchik aims to share the AI course with other programs that can serve as hosts for training. He also wants to partner with a South American university to host the course in Spanish. “The program has grown more than I could ever have imagined,” Perchik said. In March 2022, Perchik received an Education Project Award from the Radiological Society of North America, which he is using to develop a course website with recorded lectures, journal clubs, forums and research opportunities for residents and trainees. “We hope to build one of the hubs for AI education and scholarship throughout the United States and internationally,” he said.
When Perchik served as chief resident, UAB Radiology’s position as an AI leader was a useful recruiting tool. “That is something I would highlight with candidates — not only is it unique that we have this AI course, but we have hands-on experience in using these AI-enhanced programs,” Perchik said.
“If you were to go to a private practice and say, ‘I’ve used this program before; it was useful’ — or ‘We need to be a little more critical before we invest in this,’ that’s a huge benefit for our residents.”
Five ways radiologists are using AI
A screenshot of the AI Metrics platform.In his talk at the AI Literacy Course in October 2022, “The Future of AI in Radiology,” Andrew Smith, M.D., Ph.D., offered an overview of AI use cases.
1. Deep learning image reconstruction
What it is: “To get a great-looking PET scan, you have to give a full radiotracer dose and do a full scan time,” Smith said. “If you cut the radiation dose by a fourth, then you are going to get noisy-looking images. Conversely, if you keep a full radiation dose but cut the scan time to one-fourth — say from 20 minutes down to five — you are going to get similar-looking images that are noisy. Deep learning image reconstruction allows us to regain that signal by essentially reducing the image noise …. Now, a five-minute PET scan may be achievable.” There is a similar argument to be made for MRI. Sitting in an MRI scanner “is not a lovely thing to do, and some of these patients are in pain or just have other problems with their back or breathing issues, so getting through these exams quickly is a bit more important on MRI,” Smith said.
Potential benefits: Increased patient satisfaction because they do not have to spend as long in the scanner; reduced radiation dose; better scan quality. Operationalizing the time savings is not as simple as it seems, Smith notes. Scanning time is just one aspect of the imaging process; a few minutes saved may not actually generate more scans per day.
2. Computer-aided detection and triage
What it is: Algorithms can sort through images before they arrive to the radiologist and automatically move patients with positive studies or abnormal findings to the front of the queue; they may also provide important context about the patient.
Potential benefits: Identify abnormalities that a radiologist may have missed; improve efficiency by reducing the time radiologists spend locating abnormalities. Because these algorithms are not directly connected to the radiologist’s Picture Archiving and Communication System, they rely on “widgets” to pop up important information. But these can fail to catch the attention of users. “The human-to-AI interface is weak” at the moment, Smith said.
3. Natural language processing for reporting
What it is: Nearly all reports produced by radiologists include a findings section and an “impression” or conclusion section, Smith said. “You can train AI on tens of millions of imaging reports to teach the AI to automatically generate a conclusion or summary based on the text of the image findings.” In clinical practice, radiologists use talk-to-text dictation systems to detail all image findings. Once the findings are complete, the radiologist can simply click on a widget to activate AI that drafts a report “conclusion” within a few seconds. “Sometimes it gets it right; sometimes it gets it pretty close and you just need to adjust it,” Smith said.
Potential benefits: In his experience working with such tools at UAB, “it does save some time, and I think it takes a little bit of cognitive load off the radiologist,” which could help prevent burnout, Smith said. “If a report is really long and has a lot of findings, you are going to want to press that button and have it summarize those findings for you.”
4. AI and natural language processing for patient management
What it is: Detection and triage tools are generally intended to help radiologists find urgent issues that must be addressed. But AI and NLP have also shown potential for “opportunistic screening,” which Smith described as “screening for a disease passively using patient encounters or images obtained for a different purpose.” For example, there are about 100 million CT scans done in the United States each year, and about half of those are chest or abdominal CTs. Research has shown that algorithms can look through these images and detect aortic calcification (which is an early sign of heart disease), cardiomegaly (enlarged heart), aneurysms, lung nodules, cirrhosis, low bone density and other warning signs.
Potential benefits: Finding important signs of serious disease early can save patient’s health, and their lives, in some cases. “At UAB, we do 80,000 CT scans a year that are of the chest or abdomen, and an AI algorithm focused on detecting a heart abnormality could work on all of those,” Smith said. “You would direct the patients that have pathologically enlarged hearts (cardiomegaly) for an echocardiogram, an EKG and a full clinical workup.” What is the next step to implementing proof-of-concept studies in the hospital? “We haven’t worked that out in our field as to what to do with that information,” Smith said. “But some of us are interested in trying to figure that out: How do you get that information and then interact with the patients?”
5. Augmented intelligence
What it is: Smith is particularly excited about the possibilities for augmented intelligence systems — he has a startup company in this space for advanced cancer, called AI Metrics. Patients with cancer receive multiple scans over time to track tumor progression, with radiologists measuring the change in tumor size over time (or, more likely, multiple radiologists will have measured and reported on the tumors over the course of a patient’s treatment). The results determine whether their doctors continue with current treatment, switch treatments or pursue other therapies. Augmented intelligence refers to the use of AI to improve human performance. The AI Metrics solutions use AI to measure, label and track tumors over time. The radiologist is guided by the AI and smart programming. The solution automatically calculates percent changes in tumor size over time and displays the information in the form of a graph, table and key images. The improved clarity in reporting helps cancer doctors more accurately and precisely treat the underlying cancer.
Potential benefits: AI assistance improves standardization, detects and labels prior lesions, and generates reports that can often be populated at the click of a button rather than by a radiologist dictating a note (a process that can lead to errors). At any time, the radiologist can re-measure, re-write or amend the report. In a multi-institutional trial of the AI Metrics tool, Smith and colleagues found that it improves accuracy, reduces major errors (incorrectly entered data, mathematical errors, right/left errors), was twice as fast as dictation and improved inter-observer agreement by the oncologist.
The economics of AI in radiology
Screenshot from the "Economics and Ethics of AI" talk during the AI Literacy Course in 2022.In his talk for the AI Literacy Course in October 2022, Emory University’s Hari Trivedi, M.D., director of the university’s Healthcare Innovation and Translational Informatics Lab, gave a fascinating breakdown of some of the financial considerations for companies developing AI tools and the radiology departments and private practices purchasing them. The number of models cleared by the FDA is growing fast. There were 193 at the time of his talk in October — and 200 as of Nov. 10, 2022.
What are the major areas where AI models are being deployed?
- Chest CT
- Chest radiograph (X-rays)
- Brain CT
- Brain MRI
- Mammography
“Chest CT, chest X-ray and mammography are among the highest-volume studies,” Trivedi said. “Small movements or impacts in performance have large downstream consequences …. If you save 5 percent of time on a mammogram and … your institution reads 40,000 mammograms a year, that adds up.” Brain CT and MRI, used to diagnose stroke and hemorrhage, are not as high-volume; but they are very high-acuity, he added. “We see concentrations of models in areas where there is potential to have high impact.”
How much does it cost to build an AI model?
“The time and effort to develop an FDA-cleared model beginning to end is somewhere in the one- to two-year range if everything goes perfectly,” Trivedi said. And it usually costs “around $250,000 to $1 million minimum. That is before marketing and before sales and before deployment. So as ideal as it would be to create models for less common studies — for example, pediatric brain tumors — from a financial perspective, that’s not really viable right now.”
How much could an AI model save a radiologist practice or hospital?
“If you can squeeze in an extra three MRs per day, you are generating revenue on the order of an extra $10,000 per day, easily,” Trivedi said, (in addition to allowing three more patients to get their scans as rapidly as possible). Even seemingly simple AI models, which automatically straighten and align images before they are presented to the radiologist, can have a significant financial impact by increasing throughput. Or, at least, that is the sales pitch from companies marketing these products.